Accurate cell classification is the groundwork for downstream analysis of single-cell sequencing data, yet how to identify true marker genes for different cell types still remains as a big challenge. The commonly used methods for cell marker gene identification usually rely on statistical tests to search for genes that are differentially expressed between cells of interest and all other cells in a dataset. However, differential expression analysis-based methods cannot guarantee the expression specificity of identified genes in the target cells, and the commonly used methods also have shortcomings such as low computational efficiency. Additionally, with the development of single-cell ATAC sequencing and spatial transcriptomics technologies, the need for a universal method capable of identifying cell marker genes from multiple types of single-cell data modalities is rapidly emerging.
Prof. WANG Xiu-Jie’s group from the Institute of Genetics and Developmental Biology (IGDB) of Chinese Academy of Sciences, in collaboration with Prof. PEI Xiaobing’s group from the School of Software of HuaZhong University of Science and Technology, developed a cosine similarity-based method, called COSine similarity-based marker Gene identification (COSG), for more accurate and scalable marker gene identification.
The study entitled “Accurate and fast cell marker gene identification with COSG” was published in Briefings in Bioinformatics on January 19, 2022 (
https://doi.org/10.1093/bib/bbab579).
The basic concept of COSG is to compare two genes within a given cell population by evaluating the angles between the vectors representing the expression pattern of each gene in an n-dimensional cell space. Within the cell space, each dimension represents a cell. The representative vector for each gene consists of n-basis (n equals to the number of total detected cells), and the coordinate of each basis represents the gene’s expression level in each cell. Therefore, the cosine similarity of two genes equals the cosine value of the angle between the two genes’ representative vectors in the cell space. The more similar the expression patterns, the smaller the angle is. If two genes have identical expression patterns, the angle between their representative vectors will be zero, regardless of their expression abundance difference. Therefore, cosine similarity is expression scale-independent and should be more sensitive to identify genes specifically expressed in target cells. Besides, single-cell sequencing data contain many zero values, calculation of cosine similarity is very efficient on sparse matrices, which ensures the high efficiency of COSG in marker gene identification.
COSG is applicable to single-cell RNA sequencing data, single-cell ATAC sequencing data and spatially resolved transcriptome data. COSG is fast and scalable for ultra-large datasets of million-scale cells, and identifies marker genes for over one million cells in less than 2 minutes. Application on both simulated and real experimental datasets showed that the marker genes or genomic regions identified by COSG have greater cell-type specificity, demonstrating the superior performance of COSG in terms of both accuracy and efficiency as compared with other available methods.
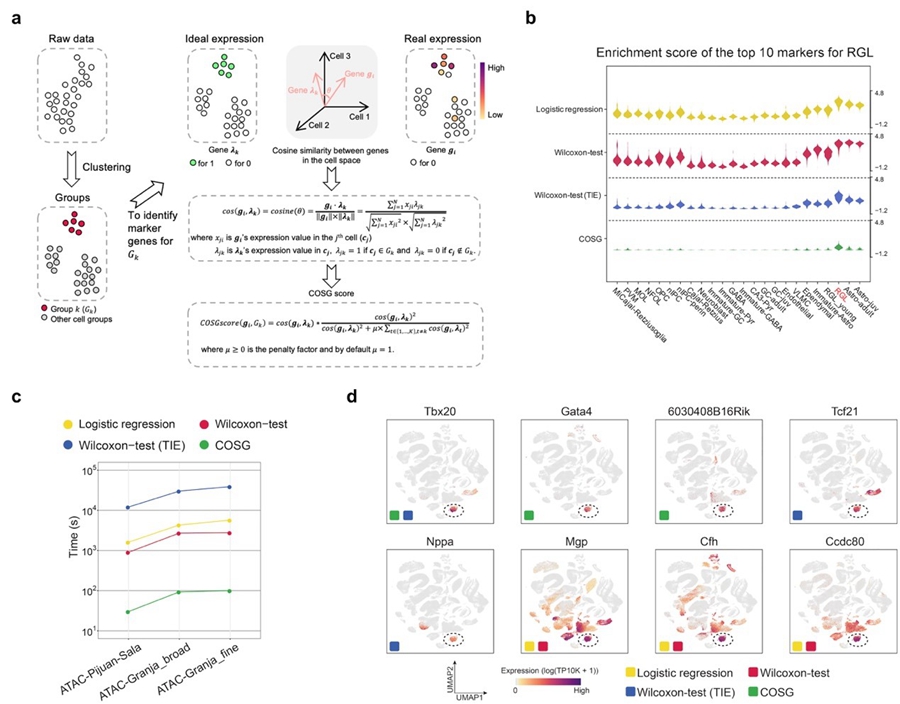
Workflow and benchmark testing results of COSG. (a) Workflow of COSG. (b) Enrichment score comparison of the top 10 marker genes identified by Logistic regression, Wilcoxon-test, Wilcoxon-test (TIE) and COSG for RGL cells. (c) Running time comparison of Logistic regression, Wilcoxon-test, Wilcoxon-test (TIE) and COSG on three scATAC-seq datasets. (d) Expression patterns of the top 3 marker genes for cardiac fibroblasts identified by Logistic regression, Wilcoxon-test, Wilcoxon-test (TIE) and COSG. (Image by IGDB)
This research was supported by grants from the National Key Research and Development Program of China, Natural Science Foundation of China, CAS Strategic Priority Research Program and Beijing Natural Science Foundation of China.
Contact:
Prof. WANG Xiu-Jie
Institute of Genetics and Developmental Biology, Chinese Academy of Sciences