In contrast to traditional cancer treatments such as surgery, chemotherapy, and radiotherapy, Photodynamic Therapy (PDT) emerges as a novel method leveraging specific wavelengths of light to activate photosensitizers (PSs). This activation generates reactive oxygen species (ROS), which effectively destroy tumor cells. PDT is known for its high targeting precision, minimal damage to the body, and reduced likelihood of resistance development. Although PDT shows promising potential in treating solid tumors, including hepatocellular carcinoma (HCC), monitoring its dynamic therapeutic effects remains challenging. Traditional methods struggle to efficiently and accurately measure cellular changes without fluorescent labeling.
To address this issue, JIANG Yuqiang's research team from the Institute of Genetics and Developmental Biology, Chinese Academy of Sciences, has incorporated deep learning technology in PDT.
Building on previous developments involving gold nanoprobe technology for efficient radiodynamic and photodynamic cancer treatment, the team highlights the significant potential of deep learning in understanding the dynamic impact of PDT on cancer cells, showcasing its important applications in cancer treatment research and paving the way for future precision medicine.
The study employs an advanced instance segmentation algorithm, Cellpose, for reliable morphological analysis of cancer cells treated with PDT. Using Logistic Growth Model Analysis, the research predicts cellular behavior post-PDT. Experimental results reveal significant changes in cancer cell morphology and proliferation capabilities following PDT treatment. Model validation further confirms these findings.
This study demonstrates that deep learning tools can extract subtle changes in target cell morphology from high-dimensional data, unveiling the complex dynamic changes in cell morphology during PDT. This not only aids in optimizing PDT parameters but also provides potential personalized cancer treatment strategies.
The study entitled " Deep Learning Insights into the Dynamic Effects of Photodynamic Therapy on Cancer Cells" was published on Pharmaceutic (https://www.mdpi.com/1999-4923/16/5/673), and it was supported by the National Natural Science Foundation of China and the Strategic Priority Research Program of the Chinese Academy of Sciences.
Typical instance segmentation results obtained by the Cellpose algorithm at different time points and laser intensities ranging from 6% (4.6 mW) to 12.5% (9.9 mW). The white lettering in the upper left corner of the image represents the relative time point, with a time format of mm:ss.sss: (A–C) 4.6 mW, (D–F) 5.4 mW, (G–I) 6.1 mW, (J–L) 7.5 mW and (M–O) 9.9 mW, respectively. Scale bars: 30 μm. (Image by Institute of Genetics and Developmental Biology)
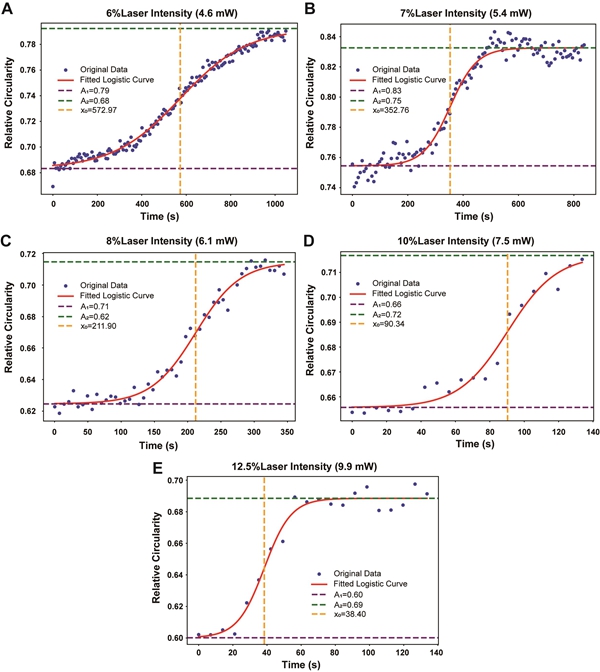
Typical dynamic profiles of cellular circularity in different groups with different PDT laser intensities. The data points, represented by blue dots, represent the observed experimental measurements of cell circularity at different time intervals. The orange curve represents the fitted logistic growth model superimposed on these data points. This model is characterized by an ‘S-shaped’ growth pattern: an initial slow growth phase, followed by a rapid exponential increase and a final plateau at a maximum limit. The results obtained under laser intensities of 4.6 mW, 5.4 mW, 6.1 mW, 7.5 mW, and 9.9 mW, respectively, are as follows: A-E. (Image by Institute of Genetics and Developmental Biology)
Contact:
JIANG Yuqiang
Institute of Genetics and Developmental Biology, Chinese Academy of Sciences